Harnessing the Power of Data in Insurance: Riding the Wave Responsibly

Written by Erik Garbin
Two massive waves are heading for the insurance industry. The first wave is the abundance of data and the advancements in analytical techniques to extract insights from it. The second is the impending wave of regulation, as legislators try to keep up with these rapidly emerging and evolving technologies.
Today, some insurers are like a surfer sitting in the water, with eyes set squarely on the horizon. They see that first wave, glassy and inviting, and start frantically paddling. They pull together the tools and data sources they need to get going, and are quickly up and riding. What they don’t realize is that one mistake, one lapse in data privacy or model governance, means they can be sure that the second wave of regulation, hidden behind the first, will come crashing down directly on top of them.
Here’s the truth: handling the shifting landscape of insurance regulations is tough. There are no one-size-fits-all rules across countries or even states. Multinational insurers have to juggle multiple privacy jurisdictions at once. And the domino-effect nature of regulators means that as certain states create guidelines, others will follow suit and potentially one-up those protections.
Recognizing this, other insurers play it safe. They limit data collection to the same questionnaires they’ve used for decades and constrain analytics to rules-based models. This conservatism may be justifiable given the uncertainty, but they recognize that a lot of value is being left on the table. They’re the surfers sitting out well past the break, resigned to letting both waves pass them by.
So, as a whole, the industry is at a crossroads. How can insurers leverage data for better business outcomes while ensuring fairness, transparency, and privacy? Let’s dig deeper into 5 ways insurers can tap into that value while balancing these two competing forces.
Collecting Data – Tapping into the Power of New Data Sources
In today’s hyper-connected world, data is everywhere. This deluge of information, spurred by the proliferation of Internet of Things (IoT) devices and wearable technologies, provides a never before seen opportunity for insurers to gain real-time, actionable insights into their customers.
Think about health insurance. Fitness trackers and smartwatches can measure everything from steps to heart rates to sleep patterns. By teaming up with health tech firms, insurers can use this data to offer personalized premiums, rewarding policyholders for staying active and healthy.
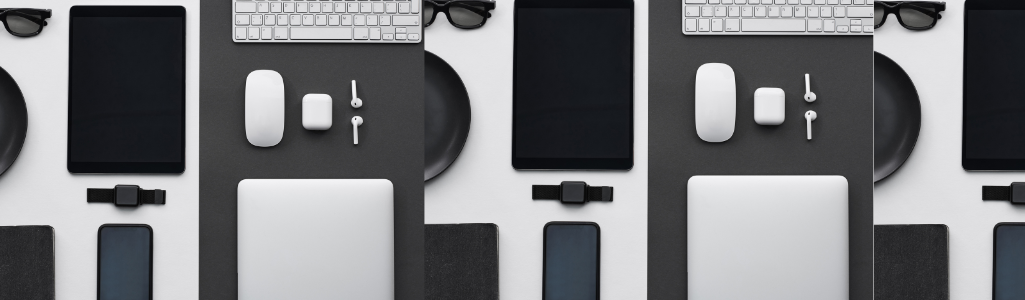
Or auto insurance. Devices in vehicles can capture speed, braking habits, and driving times. Insurers can use this to offer usage-based insurance, adjusting premiums based on real-world driving behavior. Safer drivers could pay lower premiums, encouraging responsible driving.
The theme underpinning both of these examples is a clear and transparent value exchange. Consumers get better rates, more customized products, or free devices in exchange for their explicit approval for insurers to use this data for decision making and pricing.
Regulatory scrutiny often centers around lack of transparency and insufficient consent. By making the uses consumers or clients are approving clear, insurers can avoid some of the risk that tapping into new data sources creates and keep the best interests of end-users in mind.
For insurers exploring new data sources, consider the 3 principles below:
- Make it clear what data you’re collecting.
- Explain how it will be used.
- Lay out the value consumers/users will get for providing that information.
Connecting Data – Turning Existing Data into Strategic Insights
Every customer interaction, every claim filed, every policy issued contributes to a rich, untapped wealth of data that insurers possess. But not every data set resides in a clean, structured database. Many of these data points, especially historic ones, are captured in scanned forms or handwritten notes, inaccessible in their current form.
Today’s cutting-edge tools, such as machine learning, optical character recognition (OCR), and natural language processing (NLP), offer new ways to unlock these assets and the promise of making sense of this unstructured data.
Imagine the fictional InsurCo, a 50 year old insurance company. For much of their existence, all record keeping has been analog. They have a vast pool of information – everything from a client’s initial request for quote to the final outcome of a claim – but these data points are essentially off the grid, trapped in physical documents or scanned into a PDF living on a server somewhere.
Now they decide to invest in OCR. The scanned forms and handwritten notes are digitized, turning previously inaccessible data into searchable, analyzable information. OCR scans the texts, picking up words, numbers, and symbols.
But, the story doesn’t end with digitization. Once the text is digitized, NLP steps in. This technology interprets human language, making sense of the information extracted by OCR. It discerns the context, recognizing patterns, identifying trends, and even picking up subtle nuances.
Through this process, the data from those mountains of files became dynamic, providing strategic insights that were previously impossible to attain. And, as you can imagine, this isn’t just a transformative move for their data management – it changes the game for their entire operation. They can now leverage insights from decades of data to make more informed decisions and provide a better experience for their clients.
Once data is processed with tools like OCR and NLP, it is critical to make it accessible to the teams that need it. Tools like data catalogs allow companies to centralize, tag and add metadata to these data sets, increasing discoverability and ultimately the utility of this data.
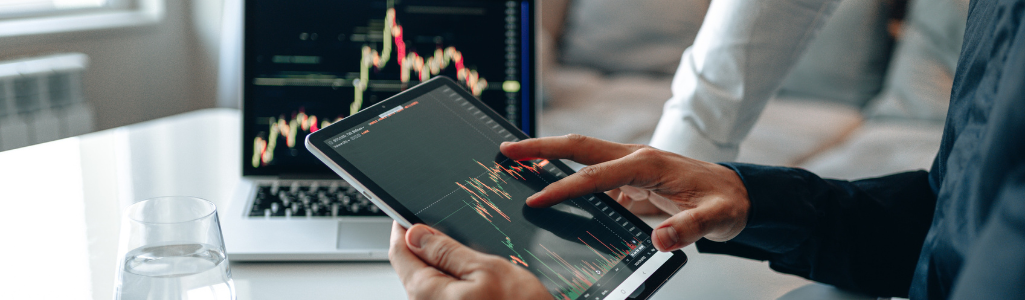
But in order to remain compliant, strict access controls must be put in place to ensure only authorized teams can access it for certain projects or purposes, and geographic restrictions from regulations or client agreements are upheld.
A few principles to keep in mind when it comes to connecting data:
- Before seeking new data sources, understand what data already exists, and to what extent it’s being used.
- Consider emerging technologies that can unlock data that was once too costly to utilize.
- Once processed, centralize and catalog data so it can be used for approved uses by authorized teams across the organization.
Data Collaboration – Unlocking the Ecosystem
Insurers are limited to the data they have access to internally or can purchase, and in some instances, the breadth and depth of that data is too small for advanced analytics. For things like fraud analytics, benchmarking, and niche product lines, models only become valuable when they’re trained on an industry wide and statistically significant number of data points. So how can individual players overcome this data scarcity challenge?
In the world of startups, we understand the transformative power of ecosystems and collaboration. The same is true in the insurance sector, which consists of a complex network of insurers, agents, brokers, Managing General Agents (MGAs), reinsurers, third-party data providers, regulators, and insurance associations. The potential upside from data sharing and collaboration within this ecosystem is tremendous, offering opportunities to streamline operations and uncover new sources of insight.
However, this potential is not without its challenges. Navigating data privacy, security, ownership, approved data uses, quality, integration, and regulatory compliance concerns can be a daunting task. Each of these elements represents a piece of the puzzle that companies must solve to harness the power of data.
Take data privacy as an example. While collaboration and data sharing can lead to deeper industry insights, companies must balance this pursuit with their obligation to protect individuals’ personal data. Similarly, while data integration can provide a more comprehensive view of a customer or risk, ensuring the quality and accuracy of combined data sets is critical.
But as is often the case in the tech world, challenges breed innovation. Emerging privacy-enhancing technologies, such as federated learning and homomorphic encryption, are providing potential solutions to the technical parts of these challenges. Federated learning allows for the training and development of machine learning models using data located on different servers without transferring it between parties, thus enabling training industry-wide models without compromising privacy. Homomorphic encryption, on the other hand, enables computation on encrypted data, allowing companies to share and collaborate on data without exposing the raw, sensitive underlying information.
Technology is only one part of the equation though. Aligning incentives between players, establishing governance and remaining compliant with regulators are all critical prerequisites for any type of coordination or data sharing, and are likely the more difficult components to implement.
By leveraging these technologies and forging strategic partnerships, the insurance industry can derive insights from data pools that would otherwise be too small or niche for an individual player to unlock. However, just as in any successful collaboration, the key is striking a balance – in this case, between capitalizing on the benefits of data sharing and upholding commitments to data security, privacy, and compliance.
When thinking about collaboration in the context of data, insurers should consider:
- Industry collaboration, if implemented in a compliant way, can help solve data availability challenges.
- Privacy enhancing technologies can form the backbone of data sharing arrangements.
- Alignment of incentives and neutrality/governance of data usage must be built in from the start.
Understanding Data-driven Decisions – Transparency is Key
Once sufficient data is sourced, connected and processed, it can be used to make decisions. I don’t need to explain here the complexity and capability of emerging artificial intelligence techniques – plenty of ink has been spilled on the dangers and promise of AI.
Despite its potential, one of the biggest barriers to adoption of AI is its ‘black box’ nature, particularly in insurance. Different sets of stakeholders need to understand how AI models arrive at decisions, and ‘we don’t know’ is not an acceptable answer to a regulator. To build trust, it’s important to deconstruct decisions and provide clear, understandable explanations of its decision-making process.
Given the inherent conservatism in insurance, many insurers tend to stick to more simplified models like random forest or boosted trees to simplify this explainability, especially for critical areas like pricing and underwriting. But insurers shouldn’t shy away from more complex and advanced modeling techniques like neural nets and deep learning. Instead, emerging technologies, like Explainable AI (XAI), can help address this challenge. XAI focuses on using algorithms that sit on top of models to drill a hole and shine a light into those black boxes, making it possible to understand and explain their decision-making processes.
Fairness and discrimination is a difficult topic with ever growing regulatory scrutiny. Everybody wants to design with fairness in mind and obvious and direct discrimination is fairly straightforward to avoid. What is more challenging is indirect bias, or proxy discrimination, where a neutral variable used in a decisioning model correlates with a protected class characteristic. This can lead to disproportionate impact, via higher rates or adverse decisions, for protected segments of the population. Therefore it’s critical to ensure both training data inputs and model outputs are tested to be free from bias to avoid unfair outcomes.
But ensuring bias-free data and decisions isn’t just a one-time effort, it requires continuous monitoring. Regular audits and robust oversight mechanisms are the key to ensuring the integrity and fairness of AI systems in insurance.
Data Privacy – A Fundamental Imperative, End to End
Privacy isn’t a feature; it’s a right. As insurers delve deeper into the data world, they must adhere to a strict commitment to privacy. Ensuring compliance with privacy regulations such as GDPR and CCPA is just the starting point. Insurers must strive to go above and beyond these minimum requirements to insure individuals are protected.
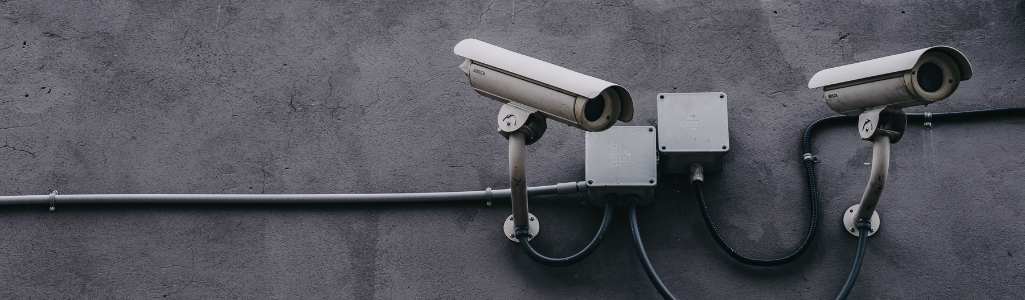
Cybersecurity is only one (albeit a large) dimension of the privacy commitment. Below are some other technologies or methods insurers can employ to uphold that commitment, ranked in order of difficulty.
- Data Minimization: Insurers should only collect data needed for specific business purposes, deleting any personal or sensitive information that is not needed.
- Data Anonymization & De-identification: This process, which involves removing personally identifiable information from data sets, can be a key strategy in protecting privacy while still allowing insurers to analyze trends and draw insights.
- Data Discovery and Transparency: Tooling to help insurers understand where data resides across their organization, who has access to it, and how it is being used.
- Synthetic Data: Utilizing artificially generated data that accurately mimics the statistical properties of real-world insurance data, without containing any personally identifiable information (PII), for model training, validation and testing.
Final Thoughts
So back to the initial question, what should insurers do in a data-driven world?
Be optimistic. The environment creates an opportunity for forward thinking insurers to differentiate amidst all this complexity. Those who can think in new ways, harnessing emerging technologies to stay ahead of regulation, don’t necessarily need to make a tradeoff between privacy and business insights. Similar to how the invention of inflatable vests and jet ski rescues helped make surfing safer on massive waves, emerging innovations like Privacy Enhancing Technologies and Explainable AI can make it safer for insurers to experiment and unlock value from their data.
In light of all of this, as insurers turn towards this data-driven future, they need to remember to balance the exciting growth opportunities with the obligations they bring. The path ahead promises a world where insurance is more personalized, efficient, and equitable. Yet, this vision can only become real if commitments to trust, fairness, and privacy are upheld.